This fictional graphic on the left illustrated ChatGPT 4.o to the prompt “create an image about the structure of your algorithm”. The magnifying glass comes from Black Forest Lab’s Flux Schnell with a real snippet of the Python code
Fairness
Hybrid Systems Reveal Biases In Learning Models
Artificial intelligence (AI) is becoming more and more prevalent in everyday life. This raises the question of how to mitigate the negative effects of this technology. Bias is a common problem in AI systems. In a recent study, a research team from L3S and Leibniz Universität Hannover presents a hybrid AI system that documents biases in machine learning (ML) models. The innovative technology can help improve the transparency and interpretability of these complex systems.
Biases in AI models can occur for a variety of reasons, as the systems are susceptible to human input during the development process. In addition, these systems are often trained on data that reflects social inequalities. Their use would therefore systematically disadvantage certain groups.
Combining AI paradigms
The authors propose a hybrid AI system that combines components of subsymbolic and symbolic AI to document bias at each stage of the ML pipeline. The system can be implemented in two ways: The first allows fine-grained tracking of biases throughout the ML pipeline; the second approach provides a broader view of detected biases in the input data and predictions.
A key element of this system is that the documentation is not only understandable by human analysts but also machine-readable. This provides the basis for better interpretability and understanding of how biases affect ML systems in a given context.
Biases in fake-news classification
The researchers evaluated their approach using a practical example based on the detection of fake news. The hybrid AI system Doc-Bias semantically described a pipeline for classifying fake news based on two benchmark datasets that used the distribution of news content, user data, and information from news publishers. The implementation of Doc-Bias then generated bias traces that reflect the inner workings of the classification system – from input to output. The authors were able to identify significant biases in the data sets that affected the predictions of the models.
Challenges with Unbalanced Data Sets
The results of this work show that even under the assumption of balanced datasets during data preprocessing, the internal processes of the AI model can compensate for attribute-oriented biases that have a significant impact on the overall accuracy and effectiveness of the fake news detection system. Specifically, the authors report a strong skew in the distribution of input variables towards the fake news label and uncover how a predictive variable leads to more limitations in the learning process. Overall, they highlight the open challenges in training models with unbalanced datasets.
This problem is not limited to fake news detection. Biases in AI systems can lead to systematic errors in almost all areas of AI application, from automated facial recognition to decision-making systems in banking.
Transparency and accountability are key
The proposed hybrid AI system is a novel technique that systematically documents the biases detected in AI models and can support human analysis in subsequent efforts to mitigate those biases. Such advances are critical to ensuring that AI systems are not only accurate but also more fair and transparent.
The authors emphasize that documenting bias is not a panacea, but only one of many steps to create accountability among AI developers and users. Socially responsible AI also requires transparency throughout the development process. Creating thorough documentation can help.
Mayra Russo, Yasharajsinh Chudasama, Disha Purohit, Sammy Sawischa, Maria-Esther Vidal: Employing Hybrid AI Systems to Trace and Document Bias in ML Pipelines. IEEE Access 12: 96821-96847 (2024) ieeexplore.ieee.org/document/10596297
Contact
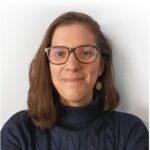
Mayra Russo, M. Sc.
Mayra Russo is an L3S PhD student in the NoBias project. She is interested in developing computational methods for documenting biases in AI systems using semantic data models. She is also interested in investigating the social implications of datafication.
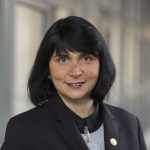
Prof. Dr. Maria-Esther Vidal
L3S member Maria-Esther Vidal is a full professor at Leibniz Universität Hannover and heads the Scientific Data Management (SDM) working group at TIB – Leibniz Information Center for Science and Technology. She conducts research in the areas of data management, semantic data integration, and machine learning over knowledge graphs.