Das Bild wurde von Midjourney für den Prompt erstellt: „Foto einer Gruppe von Studenten, die lässig in einem Raum mit einem großen Computer sitzen, alle halten Smartphones und Tablets, helle Linien zeigen die digitale Vernetzung, Schlosssymbole verweisen auf die Sicherheit.“
Fairness
Chancengleichheit beim föderierten Lernen
Modelle der künstlichen Intelligenz werden traditionell mit zentral gespeicherten Datensätzen trainiert. Dieser Ansatz birgt jedoch erhebliche Herausforderungen im Umgang mit sensiblen Daten, insbesondere im Hinblick auf den Datenschutz und die Datensicherheit. Föderiertes Lernen (FL) bietet eine sichere Alternative, indem Modelle auf dezentralisierten Daten trainiert werden, wodurch sichergestellt wird, dass sensible Informationen lokalisiert bleiben. Trotz seiner Vorteile besteht eine der größten Herausforderungen bei FL darin, ein optimales Gleichgewicht zwischen Fairness und Genauigkeit der Modellleistung zu erreichen. Wissenschaftler des Forschungszentrums L3S haben sich in einer aktuellen Studie mit dieser Herausforderung befasst und eine innovative Lösung namens FairTrade vorgestellt. Dieser Ansatz verbessert die Fairness bei gleichzeitiger Aufrechterhaltung eines hohen Genauigkeitsniveaus in föderierten Lernanwendungen und ebnet so den Weg für gerechtere und zuverlässigere KI-Systeme.
Genauigkeit und Gerechtigkeit im Einklang
Föderiertes Lernen ermöglicht das Training eines gemeinsamen KI-Modells mit Daten mehrerer Geräte, zum Beispiel Smartphones oder Tablets, ohne dass die Daten von den Geräten übertragen werden. Dieser Ansatz verbessert den Datenschutz und die Datensicherheit. Er bringt jedoch auch Herausforderungen mit sich, da unterschiedliche Daten auf den verschiedenen Geräten zu Unterschieden in der Leistung des Modells führen können. So können beispielsweise unterrepräsentierte Datensätze zu verzerrten oder ungerechten Vorhersagen des KI-Modells führen. „FairTrade zielt darauf ab, diese Diskriminierung bei Vorhersagen zu minimieren“, sagt Maryam Badar, Hauptautorin der Studie. „Durch den Einsatz von Mehrzieloptimierung versucht es, einen optimalen Kompromiss zwischen Modellgenauigkeit und Fairness zu erreichen.“ Der Rahmen lässt sich je nach den Anforderungen der Anwendung an verschiedene Fairness-Konzepte anpassen, darunter statistische und kausale Fairness.
Experimente mit realen Datensätzen aus Bereichen wie Bank-, Personal- und Gesundheitswesen haben bemerkenswerte Ergebnisse gezeigt. FairTrade verbesserte die Fairness in allen Szenarien, ohne die Genauigkeit zu beeinträchtigen. Selbst bei stark unausgewogenen Datensätzen erwies es sich als zuverlässige Alternative zu bestehenden Methoden.
Viele Anwendungsbereiche
FairTrade hat das Potenzial für eine breite Anwendung in unterschiedlichen Bereichen. In jedem Kontext, in dem KI-Systeme personalisierte Entscheidungen treffen, kann dieser Ansatz dazu beitragen, gerechtere und ausgewogenere Ergebnisse zu erzielen.
In der medizinischen Diagnostik könnte FairTrade beispielsweise Verzerrungen ausgleichen, die sich aus dem Training von Modellen mit ungleichmäßig verteilten Patientendaten ergeben. Durch die Abschwächung dieser Verzerrungen kann die Methode die Diskriminierung von Minderheitengruppen im Gesundheitswesen verringern, was letztlich zu genaueren Diagnosen und besseren Behandlungsergebnissen führt.
Ähnlich verhält es sich bei der Kreditvergabe, wo sich die Banken zunehmend auf KI stützen, um die Kreditwürdigkeit ihrer Kunden zu bewerten. FairTrade kann dazu beitragen, eine voreingenommene Entscheidungsfindung zu verhindern, die bestimmte Gemeinschaften ungerechtfertigt benachteiligt. Dies gewährleistet ein integrativeres und gerechteres Finanzsystem.
Die Forscher betonen, dass Fairness von Anfang an in KI-Systeme integriert werden muss. Post-hoc-Anpassungen der Ergebnisse reichen nicht aus, um Fairness umfassend zu berücksichtigen. „Unsere Ergebnisse zeigen, dass es möglich ist, Fairness während des Trainingsprozesses einzubauen, ohne die Leistung des Modells zu beeinträchtigen“, so Badar. Dies ist ein bedeutender Schritt in Richtung eines ethischen und fairen Einsatzes von KI-Technologien.
Maryam Badar, Sandipan Sikdar, Wolfgang Nejdl, Marco Fisichella: FairTrade: Achieving Pareto-Optimal Trade-Offs between Balanced Accuracy and Fairness in Federated Learning. AAAI 2024: 10962-10970 ojs.aaai.org/index.php/AAAI/article/view/28971
Kontakt
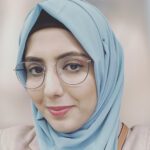
Maryam Badar, M. Sc.
Maryam Badar ist Doktorandin am L3S. Sie forscht zu Fairness von KI-Technologien.