AI, Energy and Resource Conservation: Opportunities and Challenges
In times of climate change and limited resources, the need to find sustainable, peaceful and efficient solutions is more urgent than ever. Artificial intelligence (AI) has the potential to play a key role in addressing these global challenges, for example through better climate modelling or the expansion of renewable energy. However, there is growing concern about the enormous energy consumption of AI systems themselves. Large AI models such as ChatGPT require considerable computing resources, resulting in a significant carbon footprint. A new study shows that by 2027, AI could consume as much electricity as the Netherlands in one year, i.e. around 0.5 per cent of the world’s electricity consumption. Data scientist Alex de Vries estimates that AI chips will consume between 85 and 134 terawatt hours of electricity per year by 2027.
So AI is both a problem and a solution. The L3S Research Center is therefore not only concerned with the enormous challenges of AI but also with its application potential: L3S scientists are developing solutions to make AI more energy efficient and research possible applications in the expansion of renewable energy and environmental protection.
Energy efficiency of AI systems
In particular, the training of large neural networks requires enormous computing resources and therefore a considerable amount of energy. Even training relatively small large language models consumes many times the amount of CO2 emitted by several cars over their entire lifetime. Large language models also require about 30 times as much energy for inference as a Google search query. However, there are promising approaches to improving the energy efficiency of AI.
At L3S, scientists are developing automated Green ML methods for driver assistance systems, which are installed in more and more cars. These systems optimise energy consumption by performing only the necessary calculations while maintaining a high level of accuracy. By using automated machine learning (AutoML) techniques, these approaches can later be transferred to other applications. Hypersparse neural networks are another exciting area. They aim to learn only the most relevant weights of neural networks, thereby reducing the number of operations required and thus the amount of energy needed. This requirement can be reduced even further with so-called binary neural networks, which are extremely easy to implement in hardware. In some cases, energy savings of over 95 per cent are possible without compromising on quality.
The development of energy-efficient hardware accelerators for neural networks is the focus of the L3S project ZUSE KI-Mobil. These accelerators are specially designed for use in embedded applications and help to reduce energy consumption in mobile and networked devices.
AI in use for renewable energy
AI can also contribute directly to the expansion of renewable energies. For example, the L3S project WindGISKI is developing an AI-based platform for geographic information systems (GIS) that identifies potential areas for wind turbines. The GIS platform will help identify the best locations for wind turbines, maximising the efficiency and expansion of wind energy.
Another notable L3S project is OffshorePlan, which deals with logistics for wind turbine construction sites. AI can optimise the planning and execution of logistics processes, reducing not only costs but also the environmental footprint of construction projects.
Protecting the environment and resources with AI
In addition to promoting renewable energy, AI can also make a significant contribution to protecting the environment and conserving resources. An outstanding example is the L3S project SWIFTT (Satellites for Wilderness Inspection and Forest Threat Tracking), which uses AI-supported satellite data to recognise threats to forests at an early stage and take appropriate protective measures.
The L3S project GLACIATION shows how the development of a distributed knowledge graph can improve the efficiency of big data analysis and thereby reduce carbon emissions. This knowledge graph enables large amounts of data to be processed more efficiently, helping to reduce energy consumption.
Research at L3S
The L3S Research Center works intensively at the interface between AI, energy efficiency and resource conservation. The aim is to develop new methods and technologies that both increase the performance of AI systems and minimise their energy consumption. The L3S covers the entire spectrum: from basic research into new architectures for neural networks and specialised hardware to the efficient development of new AI applications with AutoML and AI applications for sustainability.
The potential of AI for energy efficiency and resource conservation is enormous. Through the continuous further development and targeted use of AI technologies, we can not only increase the efficiency of our systems but also make a significant contribution to protecting our environment. The projects presented in this issue of Binaire provide an impressive insight into the many ways in which AI can contribute to sustainability.
Contact
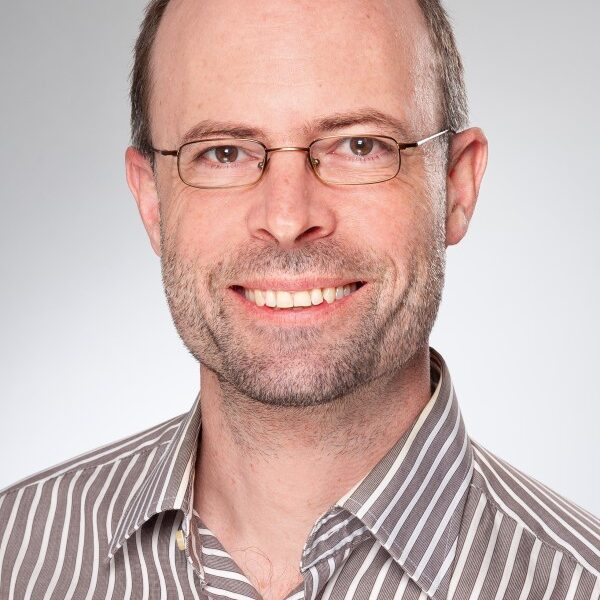
Prof. Dr.-Ing. Bodo Rosenhahn
Bodo Rosenhahn is Director at L3S and heads the Institute for Information Processing at Leibniz Universität Hannover. He conducts research in the fields of computer vision, machine learning and big data.
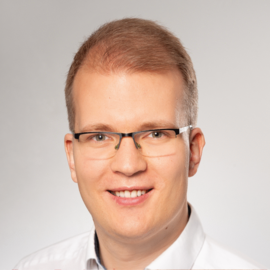
Prof. Dr. Marius Lindauer
L3S member Marius Lindauer is Head of the Institute for Artificial Intelligence and Professor of Machine Learning at Leibniz Universität Hannover.