The image is created by Flux for the prompt “three monitors with two different Electronic Health Records and a small X-ray, professional photography, bokeh, natural lighting, canon lens, shot on dslr 64 megapixels sharp focus”
AI in Medicine
Efficient Analysis of Patient Data
Electronic health records (EHRs) are a valuable source of data for medical research. They enable personalised predictions, diagnoses and treatment recommendations. Scientists at the L3S Research Center have now presented IVP-VAE, a model that enables accurate and efficient analysis of EHRs.
EHR data is often collected irregularly, meaning that measurements are taken at irregular intervals, data points are sometimes missing, and sequences vary in length. These complexities make analysing EHR data particularly challenging for existing models such as recurrent neural networks (RNNs) and transformers. “Our work shows that we can overcome these hurdles by modelling irregular time series as continuous processes and solving them as initial value problems (IVPs),” says Jingge Xiao, first author of the study.
The novel IVP-VAE model combines variational autoencoders (VAE) with IVP solvers to process time series simultaneously rather than sequentially. This parallelization significantly reduces computation time and allows the direct modelling of continuous processes. Another highlight: the reversibility of the IVP solvers means that encoders and decoders can use the same computing processes, reducing the complexity of the model.
“With IVP-VAE, we can achieve up to 40 times the speed of existing models without sacrificing accuracy,” says Xiao. On three real data sets – including the well-known MIMIC-IV data set – IVP-VAE showed consistently better results in classifying and predicting time series. The performance is particularly impressive on small data sets, which are often used in rare disease analysis.
The results mark an important step forward in data-driven health research. IVP-VAE not only provides a powerful approach for EHR data but also shows potential for other applications with irregular time series. As Xiao notes: “This model can lay the foundation for advances in personalised medicine and significantly increase the efficiency of clinical research.”
Jingge Xiao, Leonie Basso, Wolfgang Nejdl, Niloy Ganguly, Sandipan Sikdar: IVP-VAE: Modeling EHR Time Series with Initial Value Problem Solvers. AAAI 2024: 16023-16031 ojs.aaai.org/index.php/AAAI/article/view/29534
Contact
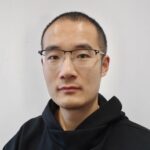
Jingge Xiao, M. Sc.
Jingge Xiao is a PhD student at L3S. His research interests include time series analysis and deep generative modelling.