©Graphicroyalty – stock.adobe.com
Helping doctors make decisions
AI in the paediatric intensive care unit
Working in an intensive care unit presents doctors with special challenges. They have to reliably detect diseases such as sepsis and make the right decisions in the shortest possible time so that the condition of their life-threateningly ill patients does not deteriorate further. In the paediatric intensive care unit (PICU), the situation is aggravated by the fact that the prevailing diseases and medical norm values vary greatly in the age group 0 to 18 years. In order to relieve doctors in this stressful situation and improve the care of critically ill children and adolescents, researchers and physicians in the Leibniz AI Lab are working on an AI-supported decision-making aid for intensive care physicians. The project is a collaboration between the L3S and the Hannover Medical School (MHH).
“We are focusing on predicting organ dysfunction using PICU data. These are, for example, vital signs such as heart rate, laboratory values such as the number of leucocytes in the blood or patient data such as age,” says Dr Zhao Ren, computer scientist and co-coordinator of the Leibniz AI Lab. The MHH provides anonymised clinical health data from more than 3,500 patients in the paediatric intensive care unit for this purpose. Artificial intelligence (AI) is to analyse the patient data in real time and support doctors in decision-making.
Training with data from health records
In recent years, huge datasets of electronic health records have been released, enabling the training of complex deep learning models for accurate temporal predictions. Nevertheless, with regard to certain patient groups or disease patterns, such as organ dysfunction in paediatrics, the available data are often insufficient and the data samples incomplete. In addition, medical time series are often irregular, as measurements are taken as needed and not for all patients at the same time. However, many models for processing sequential data assume equal time intervals between measurements. Developing deep-learning models for this data therefore poses challenges for scientists. “Based on neural ordinary differential equations, we developed an AI model that solves this problem,” says Ren. The model was first trained on a comprehensive dataset to learn features of temporal data from digital health records. It is then further refined for specific tasks, such as predicting mortality or length of stay in intensive care.
Explainablity builds trust
But why should doctors trust AI prediction? “An AI model for healthcare should not be a black box. Users should be able to understand why the AI made a particular prediction,” says Ren. This way, clinical staff should be able to understand the predictions about patients’ health, assess them and initiate the appropriate therapies. “Explainable AI enables equity, transparency and builds trust with decision makers,” says Ren. “That’s why it’s one of the fastest growing AI topics.” The use of explainable AI techniques in detecting organ dysfunction in paediatric intensive care units shows promise. For example, an AI system can visualise the measurements of vital signs whose values most influenced the prediction.
Contact
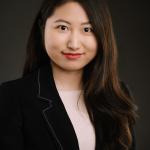
Dr. Zhao Ren
Zhao Ren was a research associate at L3S and co-coordinator of the Leibniz AI Lab.
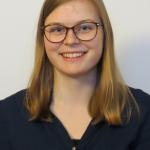
Leonie Basso
Leonie Basso is doctorand at L3S Research Center and researches for the LeibnizAILab.