©Sergey Nivens – stock.adobe.com
Generative AI for scientific knowledge
Research comparisons with ChatGPT
So now the era of generative AI has arrived – with its large language models (LLMs) like ChatGPT, which is based on the LLM GPT-3.5 with 6.7 billion parameters. Computer scientists had expected that scaling language models would boost their performance on already familiar tasks, but not that the models would suddenly be able to handle so much that was new and unpredictable. In fact, new ChatGPT usage scenarios appear daily in blogs and tweets. This phenomenon, called emergence, occurs when new complex properties or behaviors emerge from interactions within a system. Emergence is a central research question in computer science today; it has long been discussed in biology, physics or art. “As far as I know, it’s never been described in the literature that language models can do these kinds of tasks,” says Rishi Bommasani, a computer scientist at Stanford University. Last year, he helped compile a list of dozens of emergent behaviors. The Beyond the Imitation Game Benchmark (BIG-bench) project initiated by Ethan Dyer (Google Research) also documents emergent behaviors of LLMs, with 444 authors from 132 institutions contributing 204 tasks. The topics are diverse and include problems in linguistics, child development, mathematics, biology, physics, or software development, among others.
In the Joint Lab of L3S and TIB, Dr. Jennifer D’Souza and her team are working on the development and use of LLMs in the Open Research Knowledge Graph (ORKG) project. The ORKG uses semantic technologies to share research knowledge in new forms rather than PDF documents. The central element is research comparisons. They work similarly to Amazon product comparisons, except that ORKG research comparisons are based on structured, property-based descriptions of research articles. “With the Open Research Knowledge Graph, we are reinventing scientific communication,” says project leader Prof. Dr. Sören Auer.
How good is ChatGPT at comparing research articles? The scientists asked the chatbot to create a research comparison for three articles based on their titles and abstracts (Create a comparison table from the given text of the following three papers). The result: “A good first attempt,” says D’Souza.
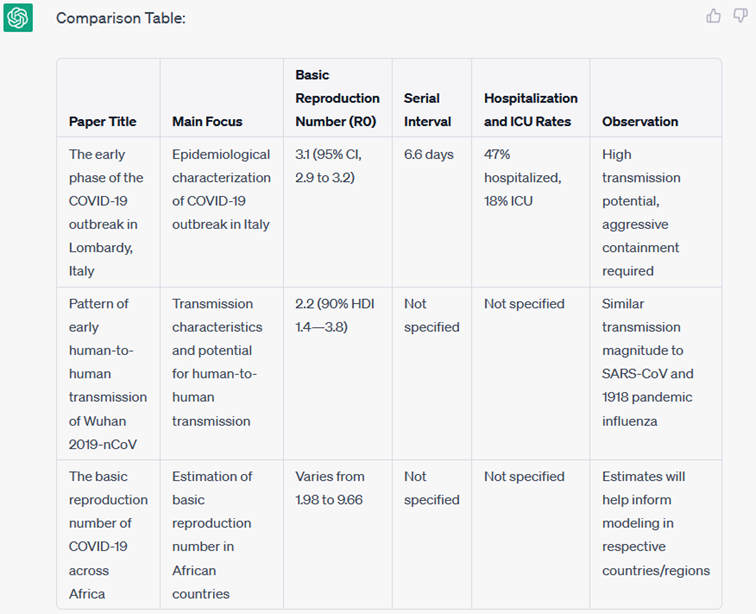
Fig. 1: ChatGPT generates a research comparison
However, an ORKG research comparison should be based on a common research problem, in this case the Covid-19 baseline reproduction number (R0). To get a conclusive overview of the contribution, the R0 number needs to be contrasted with other properties. However, the above comparison correctly reflected only one property: the value of R0. The researchers asked ChatGPT again to create a research comparison, but this time with the desired properties (Create a comparison table from the given text of the following three papers based on the following properties: location, date, R0 value, % CI values, and method).
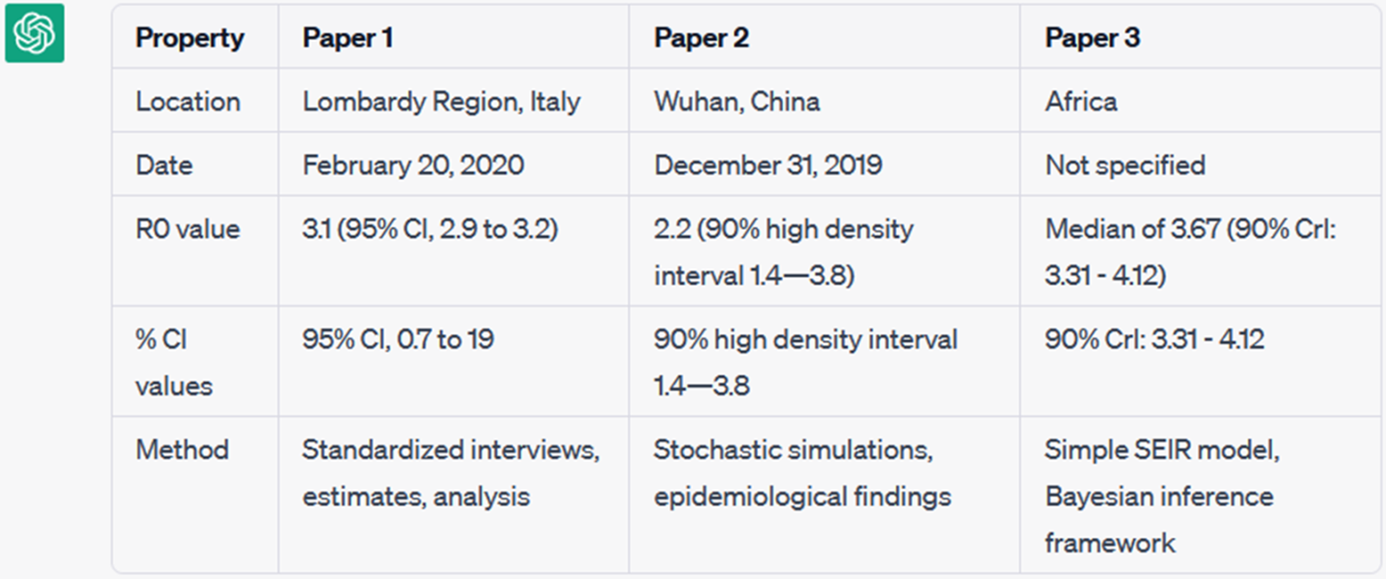
Fig. 2: ChatGPT generates a research comparison with given properties.
Bingo! The result (Fig. 2) is a research comparison that is about 80 percent correct and has no hallucinations-a phenomenon in which LLMs invent information. That LLMs generate tables is a rather surprising finding that deviates significantly from expected behavior in text generation.
In the ORKG project, the team is actively researching the use of LLMs as assistants for generating research comparisons. The article shows a positive example, but there are also cases where the model failed in the face of prolonged or insufficient context, or even hallucinated information. “Over the next few months, we plan to measure the ability of LLMs to generate research comparisons and hope to contribute to the list of emergent tasks in BIG-Bench,” says D’Souza.
Contact
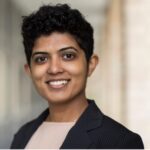
Dr. Jennifer D‘Souza
Jennifer D’Souza is a postdoctoral researcher in the Open Research Knowledge Graph project in the Data Science and Digital Libraries group at TIB. She conducts research in the areas of Natural Language Processing and Semantic Web.
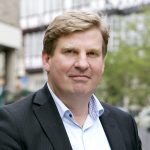
Prof. Dr. Sören Auer
Sören Auer is a member of the extended L3S Board of Directors, Director of TIB – Leibniz Information Centre for Science and Technology, and Professor of Data Science and Digital Libraries at LUH.