©The image generated by Magic Media.
Efficient and responsible AI
Slimming down neural networks
The growing use of artificial intelligence (AI) is increasing energy costs and leaving a significant footprint on the ecological balance sheet. But can the advantages of AI also be used in an energy-efficient and economical way? In the Automated Green ML for Driver Assistance Systems project, GreenAutoML4FAS for short, L3S scientists are working with the Hanover-based company VISCODA to find solutions for efficient AI applications in autonomous driving.
In recent years, new applications have enabled the public to observe how artificial neural networks have become increasingly powerful. Large neural networks such as ChatGPT require enormous computing resources. One reason for this is that they use many billions of weights. A weight indicates the significance of each individual connection between the network’s neurons. The number of weights used correlates roughly with the energy required. As future vehicles should be as economical and efficient as possible, large models from AI research cannot simply be installed in millions of vehicles. “One of our goals is therefore to compress the capability of existing large networks into small networks with few weights without significantly reducing accuracy,” says Timo Kaiser, who conducts research together with Patrick Glandorf and Prof. Bodo Rosenhahn at the Institute of Information Processing at Leibniz University Hannover.
Few weights required
Sparsified neural networks use only a fraction of the available weights. In their study Hypersparse Neural Networks, the scientists from Hanover have published a method with which regularly used weights can be successively removed from the neural network. Not only are the neural networks trained with large amounts of data as usual, but there is also an additional difficulty: the training algorithm is “penalized” if it requires too many weights to calculate the result. In order to continue solving the main task, for example classifying images, the training algorithm must restructure an already trained neural network in such a way that a meaningful classification is possible even with few weights. After training, the superfluous weights can be physically removed from the hardware. “We see that, depending on the application, up to 98 percent of the weights can be easily removed without a critical drop in accuracy,” says Patrick Glandorf, first author of the study.
New insights into AI
While investigating the algorithm, the scientists also learned interesting details about the behavior of AI. If the majority of the weights are removed before the algorithm has completed the compression, the quality of the neural network deteriorates. However, this is not arbitrary, as the example of the CIFAR 10 dataset shows. With 60,000 images classified into ten groups (cats, birds, etc.), CIFAR 10 is one of the most frequently used datasets in machine learning research. The neural networks appear to compress the ten classes to varying degrees. If compression is stopped far too early, the networks tend to distinguish between deer, birds and cats, but recognize neither horses nor airplanes. The ability to recognize the latter classes is then successively compressed during the application of the algorithm. “These findings provide information about the discrimination of certain, perhaps vulnerable groups,” says Kaiser. Horses also have a right to be recognized in road traffic.
“With our research, we are setting the course for the responsible and efficient use of artificial intelligence in everyday applications,” says Prof. Rosenhahn. “To ensure that the new technologies become widely accessible and do not become a problem for climate policy issues, we need to focus on the efficiency of AI as well as its pure ability to solve problems as accurately as possible.With the GreenAutoML4FAS project, we are taking a big step in the right direction!”
Contact
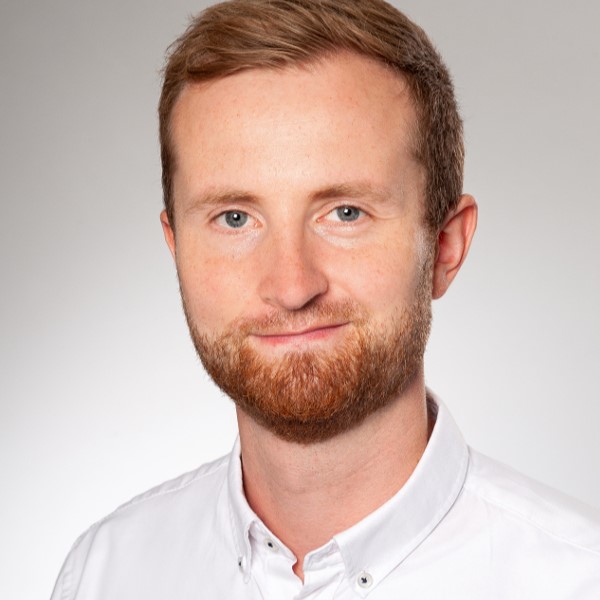
Timo Kaiser, M. Sc.
Timo Kaiser is a research assistant at the Institute of Information Processing. He works on multiple-object tracking and uncertainty in machine learning.
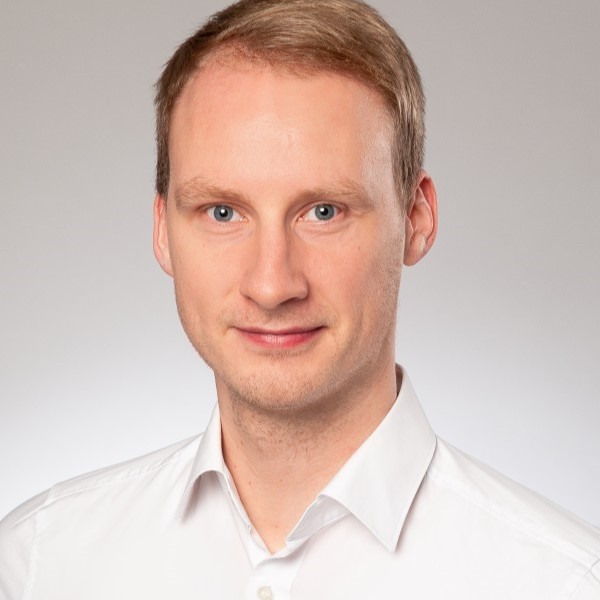
Patrick Glandorf, M. Sc.
Patrick Glandorf is a research associate at the Institute for Information Processing. He conducts research in the field of resource-efficient machine learning.
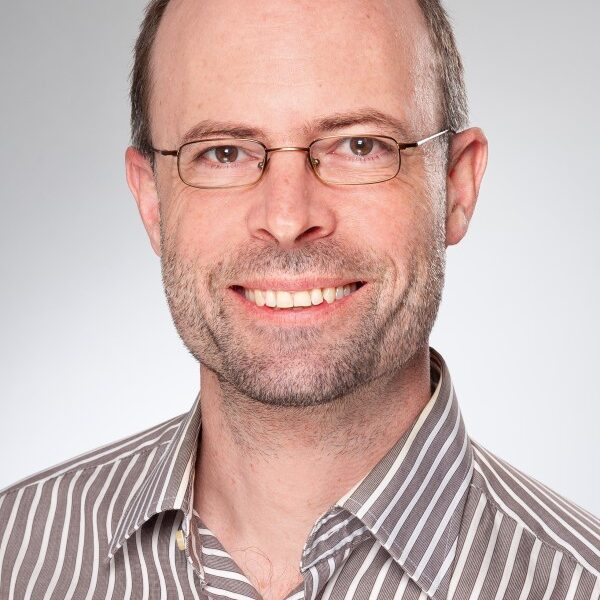
Prof. Dr.-Ing. Bodo Rosenhahn
Bodo Rosenhahn ist Direktor am L3S und leitet das Institut für Informationsverarbeitung. Er forscht auf den Gebieten Computer Vision, Maschinelles Lernen und Big Data.