The large image is created by Midjourney for the prompt ‘professional photography of flowing city traffic on a sunny morning in Germany with green traffic light’, the small one links to the prompt ‘a detailed photo of a traffic light, green, with a surveillance camera, in front of a white background, natural lighting’.
Mobility
Smarter Traffic Management
Fewer traffic jams, better public transport and lower vehicle emissions – life in the city could be a lot more pleasant. Intelligent traffic systems should provide the solution and improve traffic management. For this to work, cameras need to track vehicles, sometimes over long distances, and the data collected needs to be coordinated – a time-consuming and expensive process. Scientists at L3S have presented a solution in the prestigious Machine Learning Journal that makes multi-camera tracking more efficient: the LaMMOn AI system.
Limitations of existing systems
Existing systems are very labour-intensive. For each new camera setup, the rules for linking the recorded vehicles between the individual cameras have to be created manually. “This is very time-consuming and not very scalable,” says Marco Fisichella, research group leader at L3S and one of the developers of LaMMOn. In addition, the limited availability of public datasets makes it difficult to test and optimise new systems.
The key to greater efficiency
LaMMOn uses advanced speech and graph-based AI techniques to automatically adapt to different scenarios without manual adjustments. It consists of three main modules:
- language model detection (LMD): this module is responsible for object recognition and generates vehicle features such as type, colour and position.
- language and graph model association (LGMA): it links detected vehicles across multiple cameras and combines objects detected by multiple cameras into a global multi-camera trajectory that represents the object’s path of motion.
- text-to-embedding (T2E): This module solves the problem of data scarcity by generating synthetic object features based on textual descriptions such as ‘red station wagon’ or ‘blue SUV’.
Practical applications and successes
LaMMOn has already proven itself in several test data sets. It achieves a high tracking accuracy of over 75 per cent of the HOTA metric, outperforming many previous models.
“Our results show that LaMMOn is well suited for use in real-time traffic scenarios,” says Fisichella. With a frame rate of over twelve frames per second, the system achieves the speed required for real-time applications without sacrificing accuracy – ideal for smart cities.
The future of tracking
In addition to the technical implementation, the study highlights the role of the T2E module, which enables vehicle data to be generated from text. “This module not only reduces the effort required for manual data creation, but also makes the system more adaptable and versatile,” says Fisichella.
LaMMOn will become even more versatile in the future. The development team plans to extend the language-based functions and improve the graph structures to support even more complex applications. “LaMMOn is therefore a forward-looking solution that is perfect for traffic monitoring and control.”
Tuan T. Nguyen, Hoang H. Nguyen, Mina Sartipi, Marco Fisichella: LaMMOn: language model combined graph neural network for multi-target multi-camera tracking in online scenarios. Mach. Learn. 113(9): 6811-6837 (2024) Machine Learning Journal
Contact
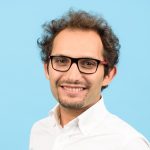
Dr. Marco Fisichella
Marco Fisichella leads a research group at L3S that focuses on artificial intelligence and intelligent systems, particularly for applications in mobility, smart manufacturing and personalised medicine.
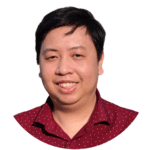
Dr. Hoang H. Nguyen
Hoang H. Nguyen was a PhD student at L3S until July 2024. Since August 2024, he is a postdoctoral researcher at the University of Tennessee in Chattanooga, USA. His research interests include graph learning, blockchain security, and transportation.