When people hear the term crystal, most of them immediately think of twinkling jewellery. In reality, crystalline materials have a much wider range of applications, for example in laser technology, optics, high-energy physics, biomedical technology or light-emitting diodes. Each area of application requires a specific type of material. New crystalline materials are constantly being tested to determine whether they have the desired properties.
Until now, it has been difficult to test crystalline materials on a large scale and to find suitable materials. All existing methods are either particularly expensive or especially calculation-intensive. Researchers at L3S have now found a method to predict the properties of crystalline materials through machine learning. The result was also published in the NPJ Computational Materials, a journal of the prestigious Nature Publishing Group.
“The published work addresses the important problem of sparse and opaque data, which are the main obstacles in predicting the properties of crystals quickly and accurately,” says Niloy Ganguly, visiting professor at L3S and leader of the study.
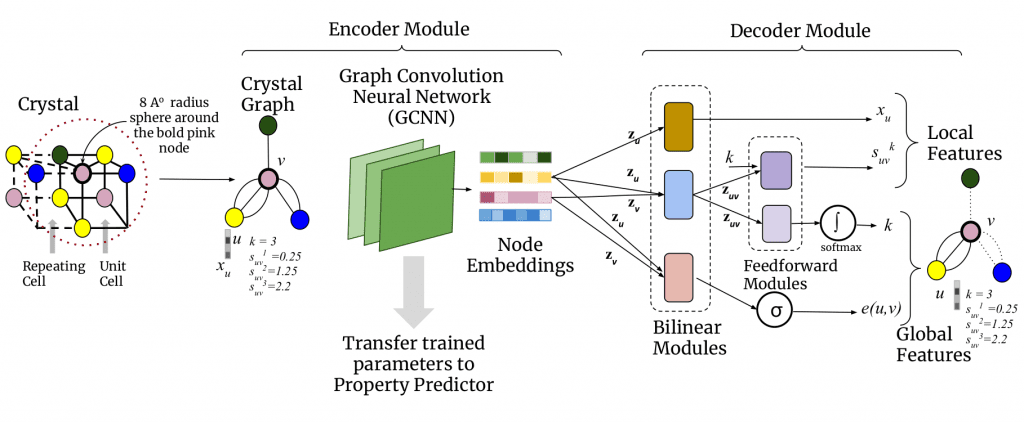
Determining the electronic, magnetic and elastic properties of a crystal is often time-consuming and expensive, as it requires extensive experimentation. Such a method is therefore not suitable for filtering out the most suitable material for a particular task from thousands of crystals. The next best method is to calculate the properties with reasonable accuracy using a theoretical method such as density functional theory, also known as DFT, instead of explicit experiments. However, this method is particularly calculation intensive.
To solve both the problem of costly experiments and that of computing-intensive theoretical estimation, machine learning (ML) methods are becoming an increasingly popular alternative. Machine learning methods are fast and do not require costly computations. However, machine learning algorithms are data-intensive, meaning they must be trained with a large amount of data from the source materials, which are labelled with property labels to accurately predict properties of new crystals. The problem: such labelled data are not sufficiently available. Moreover, the available data are not experimentally derived but theoretically calculated properties, so that training these data can lead to biases and inaccuracies in the system. With these shortcomings in mind, CrysXPP was developed, a machine learning system that enables rapid prediction of various material properties with high precision.
While property-laden data is scarce, the simple structural information of crystals is abundant. CrysXPP takes advantage of this. Because the individual atoms and their interconnections in the crystal structure are also responsible for the specific properties of the crystal. CrysXPP converts such crystal 3D structure information into 2D graphs and first learns their structural properties. Then it is trained with the small amount of available data with property labels. The first stage helps in capturing all the important structural and chemical information so that only a small amount of labelled property prediction data is sufficient to make an accurate prediction. The performance is so good that it can compensate for the disadvantage of being trained with inaccurate data sets.
Another shortcoming of ML models is interpretability, meaning that they generally cannot provide reasons for the manifestation of certain crystalline properties, which makes their use in practice unattractive and unconvincing. CrysXPP contains a selector that determines which features of the atoms are responsible for the manifestation of a certain property of the crystal.
You can find the paper and slides here.